Applying In-App Analytics to Improve User Experience and Profitability
On July 23, 2021, DELVE hosted the first of two episodes in our webinar series focused on gaming.
During the webinar, Mikalai Tsytsarau, DELVE’s head of engineering and DELVE sales executive, Adam Harackiewicz demonstrate how to use Google Cloud Platform to create a fully-integrated custom analytics system consisting of distributed data storage, scalable feature engineering engine, machine learning, and predictive modules to support a variety of business use cases.
In this webinar, we cover:
- An overview of event analytics and feature analytics (including feature selection)
- How Firebase, Firebase Predictions and AutoML can be used for segmentation and LTV prediction
- How Firebase, BigQuery and AutoML can be used for data analytics at scale
- How Red Hot Labs used in-app insights to identify high LTV users
Presenters:
Mikalai Tsytsarau, PhD, Head of Engineering
Adam Harackiewicz, Sales Executive CEE at DELVE
Over the past year, we’ve seen several disruptions beyond the COVID-19 pandemic that are reshaping data strategy and digital business. This includes Google’s discontinuation of third-party cookie support, changing privacy laws, and consumer demand for more transparency as to how their data is being used. This has accelerated the importance of collecting and integrating first-party data for targeting and customer engagement. The gambling and gaming app industry faces even more challenges to their revenue model such as a short user attention span, as 95% of mobile apps are abandoned by users within the first month.
App developers who can optimize UX and UI design for a simple, rewarding experience can increase app use — and thus, lifetime value (revenue) of each user.
In fact, studies by Bain & Company, along with Earl Sasser of the Harvard Business School, have shown that even a 5 percent increase in customer retention can lead to an increase in profits of between 25 and 95 percent.
So how should app developers simplify the user experience and increase LTV?
The answer lies in data analytics.
Data Foundation First, Data Analytics Second
DELVE’s Adam Harackiewicz, Sales Executive CEE explains that while it may sound straightforward, many roadblocks stand in the way of creating a compelling app user experience including technology complexity, lack of confidence in mobile and web data, lag times in user experience, and data silos (CRM, marketing automation, etc.)
Harackiewicz offers a client example of how his team has successfully approached data and analytics to improve user experience and profitability in the gambling and gaming industry. Stride Gaming is a successful online game operator in the UK and one of their leading revenue KPIs is identifying people that are making their first financial deposit to initiate game play. Of particular interest is the size of the initial deposit and how that correlates to future deposits and betting behavior (LTV revenue).
Harackiewicz points out that in order to increase acquisition of high-value customers through paid search ads, DELVE first needed to help Stride Gaming improve its first-party data sets to enable the application of machine learning to enable predictive LTV modeling. He says, “We were able to reduce the (search ad) spend on low value customers, and the results were that we increased the conversion rate and decreased cost of acquisition (CAC).”
DELVE offers online gaming brands a roadmap to think about how they can improve customer experience and lifetime value (profitability), by modeling their data to guide their media investments.
Watch Episode 2 of this webinar series entitled Data Science in Gambling and FTP Games: Data Enrichment.
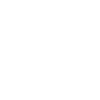
First-Party Data is Key to Segmentation, Targeting and Messaging
Harackiewicz emphasizes that everything begins with a solid customer data foundation, “this is mostly done with applications like Google Analytics, Firebase, and AppsFlyer to build the solid foundation for future models and other channels of communication.” Once the data foundation is in place, Harackiewicz says that gaming brands should ask themselves how much they are willing to pay to acquire a new customer. Finally, he says that continual improvement should be the focus, including user feedback loops.
When considering data-based segmentation, Harackiewicz offers four distinct gaming segments for brands to consider: (i) casual gamers, (ii) casual gamblers, (iii) competitive gamblers, and (iv) “long odds” gamblers. Each segment has different motivations and user experience (UX) expectations, so the gaming app should be designed accordingly. Data-based segmentation is important because it enables online game marketers to personalize messaging and experience to each segment based on their motivators and goals.
Taking a look at the buyer journey, Harackiewicz shares a six-stage model and offers an explanation of what happens at each stage: (i) awareness, (ii) acquisition & app download, (iii) trial & registration, (iv) first deposit/micropayment, (v) second deposition, and (vi) long-term usage. When you combine the four target segments with the six buyer journey stages, you end up with a table that allows you to orchestrate the messages for each segment and journey stage to progress visitors toward becoming long-term users.
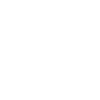
Harackiewicz also points out that once the segments are defined, lookalike audiences can be developed for further targeting with highly personalized and relevant messaging — including in-app purchases (IAP). By tailoring the gaming experience to user expectations, online gaming brands can increase usage and loyalty (leading indicators of long-term profitability).
First-Party Data is Essential for Effective Analytics
Mikalai Tsytsarau, Head of Engineering at DELVE notes that “there are many prerequisites to good analytics to establish a nice pipeline of user acquisition and to support user journeys.” He offers the first of several analytics techniques — predictive lifetime value (pLTV) modeling. He says that the goal of pLTV is “to acquire lookalike users — users that are projected to bring more value (revenue) and it’s important to change the marketing strategy accordingly, to target these high-value users as quickly as possible. This solution begins with first assembling data about user behavior, context of the user, machine learning to predict the range of projected spend, and then using it to guide user acquisition.”
One of the really useful techniques that Tsytsarau explains is predictive bidding whereby campaign bids can be set based on predicted acquisition cost and lifetime value of the acquired customer. This approach helps to immediately improve return on ad spend (ROAS). He notes that “all kinds of advanced analytics begin with the understanding of your position in the market, position among users, and the basics of user behavior. Data collection and basic understanding of trends — both external to the app and internal, are a must.”
To that end, he offers an easy-to-understand workflow journey to help online gaming brands think about the different kinds of data analytics and how they can be used:
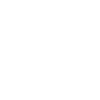
As it relates to predictive analytics, Tsytsarau highlighted that LTV and event prediction are just two types, as there are many others including churn prediction, offer prediction (for next best offer orchestration), and gameplay scenario prediction. He notes that the final step in the journey is prescriptive analytics (most advanced scenario, which attempts to propose which decisions/actions to take to prevent certain situations. For instance, once you predict churn for a user, predict the best offer and timing to maximize results. Finally, Tsytsarau recommends that online gaming brands build their analytics workflows to align with the sequence of this analytics journey.
The Basics of Machine Learning and Feature Engineering
Many people think that machine learning is magic where you throw algorithms at mountains of data to yield answers. But in reality, machine learning (ML) is a science that requires careful planning and execution.
Before diving in, Tsytsarau reminds us of some basics such as automating data collection to avoid manual processes and free up time for more data analysis and modeling the data. When building models, make sure they can scale to handle seasonality and other increases in traffic. He notes that not many companies think about this until they experience user traffic spikes. Training multiple models with multiple parameters can be straightforward with the right tools. However, one thing he stresses is that it is cyclical/recurring as ongoing optimization is key.
A simple workflow diagram is illustrated here:
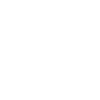
Beyond machine learning, Tsytsarau introduces the ability of using event analysis in Google Analytics. There are two types of analytics: event analytics (events of different kinds) and feature analytics (events of the same kinds) to look for patterns among users. Funnels are used as a framework for analyzing user behavior across steps in the funnel and understanding probability of performing an action based on a prior action. Additionally, Firebase is a platform for app development (backed by Google) that provides database, analytics and messaging — and it can even make advanced predictions.
When combined with tools like BigQuery and AutoML, online gaming brands can unlock serious insights from their data.
The Bottom Line
Online gambling and gaming brands can collect and structure data to reveal powerful insights to improve customer acquisition, customer experience, and customer lifetime value. By applying descriptive and predictive analytics, brands can translate trends into actionable insights. By applying machine learning and feature engineering, brands can optimize game features and improve segmentation.
For a deeper dive into data analytics for optimizing UX and LTV in online gaming, watch the second episode of the webinar series focusing on data enrichment.